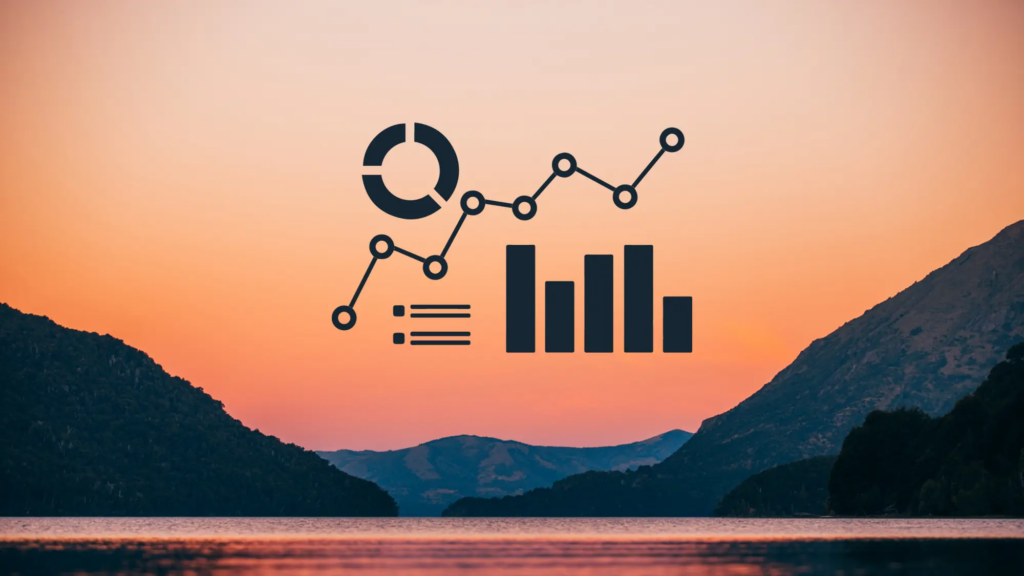
Over the past couple of decades, the importance of data has multiplied exponentially. More industries are relying on data analysis for decision-making, and those that don’t are being left behind.
However, on average, more than 80% of business data is unstructured and unsuitable for decision-making. This is where the data discovery process comes in.
The data discovery process helps businesses extract meaningful information by classifying, organizing and analyzing raw data. Having accurately analyzed data allows you to make better data-driven decisions, identify data risks and comply with data protection regulations.
This article will help you understand key stages of the data discovery process, explore tools for data discovery and explain how to measure data discovery success.
Let’s get started!
Key Takeaways
Data discovery helps you classify and report business information in an easy-to-understand format for data-driven decision-making.
Data discovery helps with decision-making, cleaning data sources and ensuring regulatory compliance.
You can use automated discovery tools, but it’s crucial to ensure alignment with your data governance policy.
What is Data Discovery?
Understanding the Data Landscape The Data Discovery Process (1).png
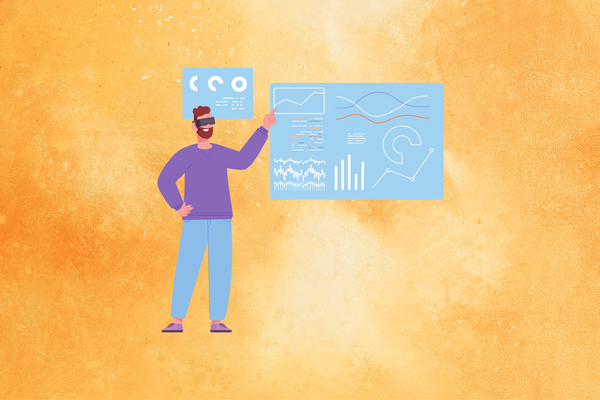
Data discovery is the process of collecting, classifying, and extracting meaningful information from raw data. The data discovery process can help you easily analyze data for accurate decision-making.
Let’s say you’re looking to identify buying patterns for next month’s marketing strategy. The data discovery process will organize data from different databases, extract only the data specific to your goal (consumer buying patterns), and organize the data using visual tools to simplify the decision-making process.
Quite useful, isn’t it?
But data discovery isn’t just important for analyzing trends and decision-making. It also helps with data management, allowing you to catalog and classify sensitive business data. Think of it as a type of enlightenment process where you can improve transparency, clean out irregular data, and manage databases with more accuracy.
What’s more, data mapping is often a prerequisite for data mapping. It helps clean out and identify which data you need to map. Considering the scale of data that businesses have to deal with nowadays, having a neat set of databases to map out can reduce the chances of mapping errors.
The Importance of Data Discovery
Ok, so data discovery is about helping with data mapping and filtering out raw data. But you’re probably thinking, why is this process so important?
Well, for one, we live in an age where information is power. Imagine you could have more accurate sales forecasts, identify potential blunders before they blow out of proportion, and get an accurate analysis of market conditions.
Data discovery helps you do exactly that.
More importantly, it helps filter data in a way that you’ll be looking at information instead of raw data. So, placing more importance on the data discovery process can give you a significant advantage over competitors, and you’ll have more meaningful, filtered data.
Supporting Data Governance and Compliance
Data discovery is also crucial for supporting data governance and achieving regulatory compliance. In today’s age, where ensuring corporate compliance is a challenge for businesses, having accurate, filtered data can give you an edge.
Most compliance frameworks require you to have transparent data systems and know exactly what type of data is stored and how it relates to your business’s goals. And thorough data discovery is a meaningful way of achieving these goals.
Enhancing Decision-Making
According to a study by PWC, businesses that rely on data for decision-making are 3 times more likely to see an improvement in the decision-making process. However, for this, you’ll need data that’s filtered according to your business’s goals.
So, if your business doesn’t have a thorough data discovery process, you’re walking in the dark, and it’s only a matter of time before you make a blunder based on inaccurate, raw data!
Key Stages of the Data Discovery Process
Understanding the Data Landscape The Data Discovery Process (2).png
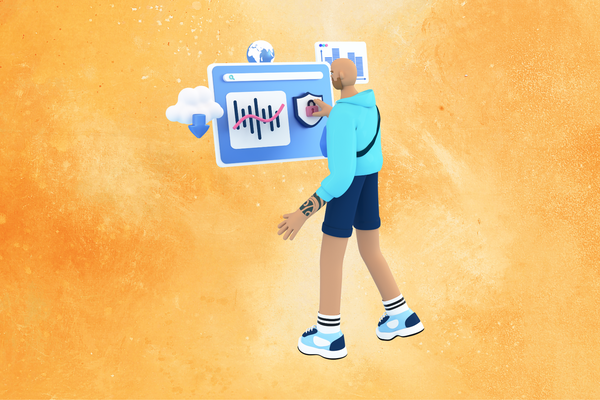
Data discovery is an ongoing process that aims to extract meaningful information and present it using visual tools for better decision-making. But, before getting to the presentation part, you’ll have to go through the whole process.
Let’s look at the key stages of the data discovery process:
Data Identification and Inventory
Before you can begin any analysis, you’ll need to know which data to classify, which sources to target, and how these link with your business’s goals. For this, you’ll need a catalog of data sources, the type of data these sources record, and the purpose of the source.
It’s crucial to get this step right, or you’ll miss out on crucial data that could distort your analysis. Most businesses use data classification tools to identify and create a catalog of data sources. This way, you’ll save time in this step of the data discovery process.
Data Profiling and Analysis
Once you have a list of the data sources, you can move on to profiling and analysis. Profiling is also called data cleansing and involves creating summaries of data. This helps you:
Gauge the data’s quality.
Understand data relationships.
During this step, you’ll be evaluating data based on accuracy and consistency and whether it meets your standards.
A simple example is classifying consumer data based on address fields and buying patterns and ignoring irrelevant fields like purchases that have chargebacks attached to them, etc. This step forms the crux of data discovery and the basis for any visual representation.
Data Visualization and Exploration
If you’ve gauged the data quality and analyzed primary sources, it’s time to start the visualization process.
Data visualization involves creating graphs, charts, and other visual tools to represent the data in an easy-to-understand manner. This process may also include data mapping, which helps visualize data flows, giving you a picture of where data is being used in your business.
Data Classification and Prioritization
Once you have the visual tools in place, you can now move towards classifying the data and prioritizing which data values to target or analyze.
Let’s look at each aspect separately:
Data classification helps you organize data summaries and tells you which categories the discovered data falls under.
Data prioritization gives value to these categories based on their importance. For example, customer retargeting data may be more valuable in a marketing campaign than raw consumer analysis data.
Collaboration and Stakeholder Engagement
The data discovery process doesn’t end with classifying and visual analysis. After all, what’s the purpose of classifying data and creating visual schemas if you aren’t using it for decision-making?
This is where collaboration comes in. You can share the visual schemas with cross-functional teams and use them for stakeholder engagement. This makes the data relationships much easier to understand and helps with better communication and feedback.
Tools and Technologies for Data Discovery
Understanding the Data Landscape The Data Discovery Process (4).png
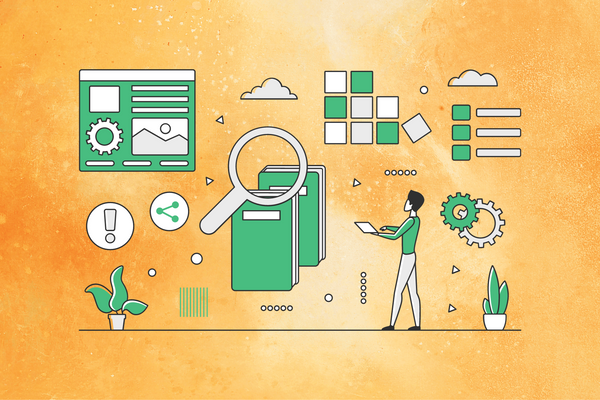
With the sheer volume and complexity of data that businesses have to deal with nowadays, it’s extremely difficult and time-consuming to do manual data discovery. Imagine sifting through Excel sheets with thousands of data entries, each linked to several sources!
Fortunately, you can easily access tools to assist with the data discovery process. These tools are designed to automate parts of the data discovery process, ensure accurate preparation, and prepare visualized reports that make it easy for non-technical stakeholders to understand.
If you really want to automate the data discovery process, try getting a tool with AI and machine learning integrations. These tools allow for real-time analysis and can accurately categorize data, do data prioritization, and grade the relationships between datasets.
Here are some popular cloud-based data discovery tools:
ManageEngine
ManageEngine is a data management system that allows you to do data discovery with a focus on security. It’s ideal for tech companies and large businesses and is perfect for compliance with GDPR, CCPA and other data privacy regulations.
Informatica
If you’re looking for a more complete tool for data discovery, Informatica is a one-stop shop. It includes tools for data discovery, extraction, information life-cycle management and other data analysis and classification features.
What’s really nice about this tool is that it automatically uncovers insights and taps into business intelligence.
Challenges in the Data Discovery Process
Data discovery sounds simple, doesn’t it? Simply integrate a tool with your data management system and get clean, visualized data.
Unfortunately, data discovery isn’t as easy to implement as you may think. And without a competent data compliance solutions provider to set it up for you, you’ll likely run into the following challenges:
Data Complexity and Volume
Most businesses have no idea of how much data they deal with every month. When classifying data, you’re probably looking at major metrics, like profitability, costs, consumer signup data, and other easily identifiable sources.
But the reality is that modern businesses have to deal with much more complex data sources. To add to the complexity, each data source may have several thousands of entries each month, leaving even small and medium-sized businesses with colossal amounts of data to analyze.
Ensuring Data Privacy and Security
Another major challenge that you’ll face with data discovery is ensuring data privacy. Data privacy laws, like the EU’s GDPR, require businesses to keep records of certain consumer data. This should be part of your accountability framework and data discovery process.
The problem isn’t integrating data discovery with data privacy regulations – that’s easy to do. The challenge is staying ahead of data privacy and security changes and keeping your business systems updated accordingly.
Data Silos and Fragmentation
The hardest challenge you’ll face with data discovery is dealing with fragmented data and data silos. These are isolated data systems with no connection with other databases, which makes them very difficult to classify.
Fortunately, the latest mapping tools can help arrange isolated and fragmented data and some AI tools can determine the relationship between these data sets and other databases.
Resistance to Change
There’s a common saying amongst business mentors: “Our only security is our ability to change.” Unfortunately, most people feel secure doing exactly the opposite.
When you hear about AI integration in data discovery tools, what comes to mind? Data security or a fear of data breaches? Or the thought of faster, more accurate data processing?
Like most modern business challenges, tools are constantly being developed to help you adapt. If you take an open-minded approach and adopt these tools in your business, you’ll easily overcome most data discovery challenges.
Measuring the Success of Data Discovery
Understanding the Data Landscape The Data Discovery Process (3).png
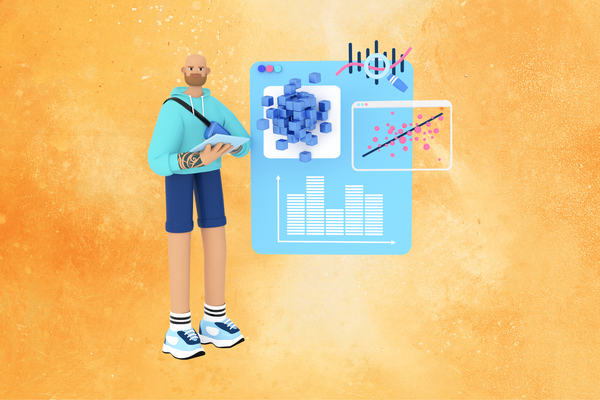
Since data discovery is linked to data mapping and other processes, most businesses make the mistake of not evaluating the process. Do so for long enough and failings in the process will affect data mapping and other related processes.
The best way to measure the success of data discovery is to create a set of KPIs to test the system against. This is usually done by data audits conducted by data protection compliance services.
Also, remember that no matter how solid your data discovery process is, you’ll need to be flexible and adapt to changes in the data discovery environment. This calls for regular audits, constantly updating your data discovery process, and reevaluating KPIs accordingly.
Data Discovery Best Practices
Data discovery is easy enough if you have automated tools, but you’ll still need to follow best practices to integrate them successfully. The last thing you want is cracks in this process, which may cause data breaches, incomplete analysis, and regulatory non-compliance.
Here are some best practices for a smooth data discovery process:
Filter Out Irrelevant Data
Data quality is crucial if you want accurate data mapping. If you don’t filter out irrelevant data before doing data analysis, you’ll end up with distorted information. In some cases, this may have a catastrophic effect on your business’s decisions.
Let’s say you have to analyze data of customers who purchased something from your business. Now, assuming your database contains data on customers who have made purchases and those who returned items.
These “returns” don’t really count as a purchase from a revenue point of view, and if you don’t do proper data discovery, you’ll end up considering it in the analysis.
See how important filtering out irrelevant data is?
Focus on Automation
Data discovery isn’t impossible without automation, but it’s certainly going to give you headaches! Even small businesses nowadays have to sift through a lot of data for simple decisions. Doing this manually could take ages, while automated tools can do it in minutes.
Automated data discovery is also safer and more accurate, especially when dealing with hundreds or even thousands of datasets.
Put Data Governance First
Data governance should be the base of any data discovery process. It lays down the principles and gives you direction on what to include in your analysis.
Data governance is closely aligned with your business’s privacy policy and the privacy regulations your business falls under. For this, you’ll need expert compliance services to create a governance policy that aligns with the relevant regulations.
Final Thoughts
Data discovery lays the foundation for data mapping, analysis, and other crucial functions. With automated data discovery tools, you can get more accurate analysis for data-driven decisions.
However, setting up a data discovery process requires regulatory expertise and an understanding of data governance principles.
At Captain Compliance, we offer compliance solutions that include setting up your business’s data discovery process, integrating automated discovery tools, and linking other processes like data mapping and more.
Get in touch today for your data discovery needs!
FAQs
What are the Three Methods of Data Discovery?
The three methods of data discovery include manual, semi-automated, and automated data discovery. Manual data discovery is a thing of the past, and most businesses will need automated tools to simplify the process.
Check out a related article on assessing data risks.
What are the Components of Data Discovery?
The main components of data discovery include preparation, visualization, and analytics. However, you may also include evaluation and reporting in this process.
Learn more about e-discovery and how it’s similar to data discovery.
What is a Data Discovery Tool?
A data discovery tool is an automated system that classifies, groups, and analyzes large amounts of business data. These tools are often connected with a data management system and some can provide real-time data discovery.
Explore data privacy acts to understand the importance of data discovery.
How do you Conduct Data Discovery?
To do data discovery, you’ll need to identify data sources, list them according to your business needs, clean and analyze the data, and report it using visual representation. You’ll also need to ensure the process covers your data privacy governance program.